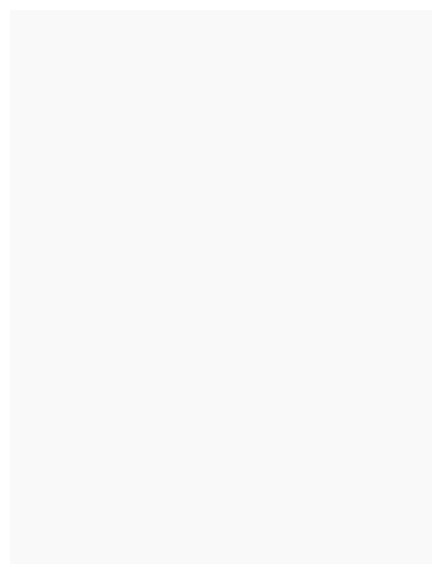

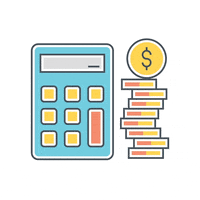
Precision in Financial Analysis
iRM employs advanced algorithms for meticulous analysis of extensive financial data, uncovering patterns and detecting anomalies.
iRM employs advanced algorithms for meticulous analysis of extensive financial data, uncovering patterns and detecting anomalies.
The latest iRM release redefines insights and transparency, providing stakeholders a comprehensive understanding of vendor risk, payroll irregularities, and expenditure trends.
Through refined entry details and cutting-edge visualization, the latest release empowers finance professionals to unravel data intricacies. This not only builds trust with contextual risk scores but also guides users in making well-informed decisions based on AI-generated insights.
iRM's cutting-edge technology employs advanced algorithms to analyze extensive financial data, identifying patterns and detecting unusual transactions or discrepancies. This empowers auditors and financial experts to assess control risks with greater efficiency and accuracy, with a specific focus on the highest-risk aspects of the business.
In our latest iRM release, we've elevated insights and transparency, providing stakeholders—customers, investors, regulators, and employees—with a deeper understanding of vendor risk, payroll irregularities, expenditure trends, and more. Building trust and ensuring comprehension of AI-generated results are critical for financial professionals, addressing key aspects:
✔ Risk Management: Financial professionals utilize AI models for predicting and managing risks. Understanding the rationale behind AI-generated insights is crucial for informed risk mitigation decisions and validating the robustness of the model.
✔ Trust and Confidence: Explainable AI fosters trust among stakeholders. When finance professionals can clarify the reasoning behind AI-driven decisions, they can confidently endorse and convey these decisions to customers, investors, regulators, and employees.
✔ Model Enhancement: Grasping the analysis allows finance professionals to identify areas for model improvement and fine-tuning, ensuring continuous optimization.
✔ Visualization Techniques: Visualizing acquired features or patterns provides insights into the model's behavior, facilitating the understanding of data point relationships.
✔ Feature Attribution: Assigning significance to each feature aids in comprehending the underlying data structure. Techniques like Local Interpretable Model-agnostic Explanations (LIME) explain the model's behavior by approximating it with a locally interpretable model.
✔ Prototype-based Methods: Identifying representative examples or prototypes captures learned patterns, facilitating a better grasp of the model's behavior.
In our latest release, we've expanded entry details, employing visualization techniques to help users comprehend data relationships. This feature contextualizes assigned risk scores, guiding finance professionals in taking appropriate actions based on AI-generated information, instilling trust in the results.
iRM's advanced algorithms enable financial professionals to predict and manage risks effectively. Understanding the rationale behind AI-generated insights is crucial for making informed risk mitigation decisions and validating model robustness.
iRM employs various approaches, including visualization techniques, feature attribution, and prototype-based methods, to amplify interpretability. These methods facilitate a better understanding of the model's behavior and data representations.
Explainable AI ensures transparency, fostering trust among stakeholders like customers, investors, regulators, and employees. Finance professionals can elucidate the reasoning behind AI-driven decisions, instilling confidence in the outcomes.
The latest release encompasses expanded entry details with visualization techniques. This feature contextualizes assigned risk scores, assisting finance professionals in comprehending data relationships and guiding them in taking appropriate actions based on AI-generated information.